![[object Object]](/_next/image?url=https%3A%2F%2Fcdn.sanity.io%2Fimages%2Fei1axngy%2Fproduction%2F7ccf7c4c80275f57d55d0bd24bcbf17b12dccdc5-450x450.jpg&w=256&q=75)
by Michelle Fabienne Bieger
Updated 24 April 2023
Ethics and Machine Learning
![[object Object]](/_next/image?url=https%3A%2F%2Fcdn.sanity.io%2Fimages%2Fei1axngy%2Fproduction%2F7a9ef0b1bf7adc4c599d9537216a349145869313-800x639.jpg&w=2048&q=75)
Machine learning and ethics are necessary bedfellows in order to ensure that new, groundbreaking algorithms can be used equitably and do not tip the scale of ethics and technology inappropriately. The real world implications of technology that hasn't been properly regulated or thought about critically can be wide-reaching and deserve careful consideration.
This article will break down some of these implications and provide practical, implementable advice for both academics and businesses to engage their agency in this important issue.
Why should stakeholders in machine learning care about ethics?
The proliferation of data about consumers and their behaviours from ever-cheaper sensor hardware and softwares make machine learning algorithms much more accessible and accurate than ever before, and thus, increasingly ubiquitous in industry sectors around the world. The effects of this can be thought of as a sliding scale of ethics and technology.
On the lower end of the scale, there are events such as replacing a customer service representative with a chat-bot until a certain amount of time or other action trigger is enacted. This type of technological intervention can be somewhat mitigated with effective government regulation, and for most citizens can represent, at most, a minor inconvenience in the flow of their day.
As the scale moves towards the other end, increasingly complex effects with much more significant societal ramifications are at stake: for example, influencing voting patterns and thus being able to control or rig a country’s national election.
To avoid tipping the scale towards that end, machine learning engineers and companies must be committed to learning more about ethics, and instilling in their workflows opportunities to catch inappropriate uses of data and violations of consumer privacy.
A case study: the OpenAI release of ChatGPT
OpenAI’s release of ChatGPT and the image-generating counterpart Dall-E serves as a case study in why companies must be alert to the potential ramifications of their products. ChatGPT, Dall-E, and their counterparts from other companies have led to massive concerns amongst a broad spectrum of stakeholders; from everyday citizens and journalists concerned about the value of the written word and intensified disinformation campaigns, to academics and educators grappling with how to refresh pedagogical standards in a new information landscape, to writers and artists fighting for recognition of their intellectual property.
The numerous fallibilities of ChatGPT, including, but not limited to: falsifying historical record, creating incorrect academic and book citations, ‘hallucinating’ scientific concepts, discriminating and showing bias, and violating GDPR regulations, have led to real-world consequences that creep towards tipping that scale of ethics and technology.
OpenAI must not only address these fallibilities, but also their exploitative labelling practices that enable ChatGPT and Dall-E’s release. In order to label not-safe-for-work content and avoid these cropping up in their tools’ outputs, OpenAI outsourced the labelling of trauma-inducing content to Kenyan labourers earning less than $2 an hour in order.
Though they were promised therapy to deal with enduring long-term effects of this kind of work, this support never materialised. This is an ongoing and common labour practice that typically exploits workers in the Global South for companies that are predominantly headquartered in, and provide services for, the Global North.
OpenAI's hesitance to address exploitative labor practices and engage in constructive discussions about the various concerns surrounding their tool stems from a startup culture that thrives on limited government oversight, prioritizes rapid market gains, and incorporates detrimental philosophies like longtermism into their corporate values, which tend to overstate AI's potential while downplaying human rights.
Yet OpenAI is not alone in their inability to cope with these root issues: AI image generator Stable Diffusion has used image sets to train their model that included sensitive medical data photos. Roomba has released nude photos of a woman in her bathroom. Tesla Autopilot has recorded an increase in fatal crashes, prompting an investigation from United States federal authorities. Whilst human judgment is not infallible, it is clear that relying on artificial intelligence disguised as machine learning techniques is not a viable sole alternative either.
From case study to lessons for start-ups
Warning symptoms of these issues can be found when analysing companies like these that violate worker’s rights and consumer’s privacy. Inadequate terms and conditions and data policies are a common symptom, purposefully obfuscating or misleading policies blind consumers to the reality of where and how their data is being handled.
Pushing products to market due to corporate stakeholder’s pressure is another, as well as en-masse firing events (particularly of employees charged with establishing ethical guidelines) before product deployment, overhyping products and clever use of psychological tactics to create an image of artificial intelligence, and exploitative use of outsourced labour.
We can use these symptoms as early warning signals of potentially unethical practice occurring at a company, and then leverage both consumer choice and government regulations to proactively make a change in the landscape of machine learning companies for the better.
Key actions to further ethical machine learning
Both individuals and companies as a whole have a responsibility to act ethically and provide a better future for the citizens they serve.
Academics and machine learning engineers need to be engaging in knowledge exchange in order to allow space for citizens to learn about machine learning and the ethics thereof. Outreach, demystifying how machine learning works to the public, and engaging with policy to ensure government regulations are kept up to date as much as possible with the quick-moving industry landscape are essential tools that can help provide momentum to social tipping points on issues surrounding machine learning.
As part of this, it is essential to understand that language and philosophy matters, and that interdisciplinary work needs to be embraced. Algorithms cannot “create” or “own” or “make”; they do not have personhood. Laissez-faire use of language like this, or philosophies that do not centre human rights and the human experience, can allow both individuals and companies to fall prey to overhyped marketing and user-interfaces.
Interdisciplinary work with linguists, philosophers, and machine learning ethicists can provide insights that prevent unethical practices or tools. Additionally, when working to solve a particular issue with a machine learning tool, it is essential to engage with the community for which that issue needs to be solved. This interdisciplinary research can not help to avoid ethical pitfalls, but build a better tool overall.
Open-access papers and code are another essential way in which individuals and companies can engage with their agency. Ensuring that science and code remains reproducible and inheritable is a key cornerstone to maintain trust in that science and code; benchmark testing and peer-review needs to be embraced in these spaces.
Finally, considering the use of ethics review boards, similar to those in the field of medical science, could be an essential tool for universities and companies to safeguard against ethical and moral concerns regarding the development of any particular machine learning application.
Ethics at digiLab
At digiLab we are looking forward to including a section on ethics in our teaching material, as well as establishing an ethics review board for our own activities, so that we can play our part in carving out a space for ethical machine learning. This article is also the result of a machine learning ethics seminar, of which we will be having more of as the machine learning landscape changes and provides more case studies from which we can learn about unethical practices.
Resources that those interested in ethics can look into include:
- The Ada Lovelace Institute report Looking before we leap: Expanding ethical review processes for AI and data science research
- The Turing Way: A Handbook for Reproducible Data Science
- An archive of discrimination and bias showcased in large language models, along with societal impacts, from researcher Emily M. Bender
- The Age of Surveillance Capitalism: The Fight for a Human Future at the New Frontier of Power by Shoshana Zuboff
- Privacy is Power: Why and How You Should Take Back Control of Your Data by Carissa Véliz
In what ways have you found machine learning, or data privacy, has changed your life, for better or for worse? How do you think the fabric of our society will change with tools like ChatGPT? Do you think that government or societal forces have enough power and agency to push back against unethical company practices?
Featured Posts
If you found this post helpful, you might enjoy some of these other news updates.
Python In Excel, What Impact Will It Have?
Exploring the likely uses and limitations of Python in Excel
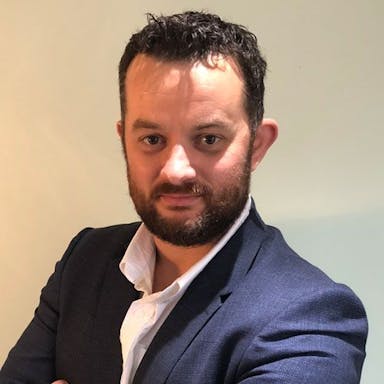
Richard Warburton
Large Scale Uncertainty Quantification
Large Scale Uncertainty Quantification: UM-Bridge makes it easy!
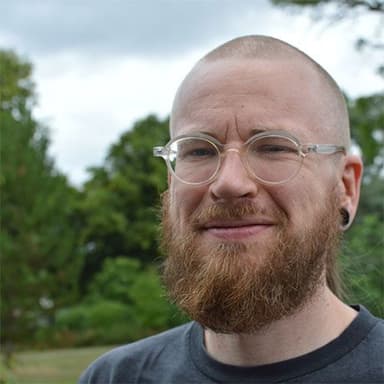
Dr Mikkel Lykkegaard
Expanding our AI Data Assistant to use Prompt Templates and Chains
Part 2 - Using prompt templates, chains and tools to supercharge our assistant's capabilities
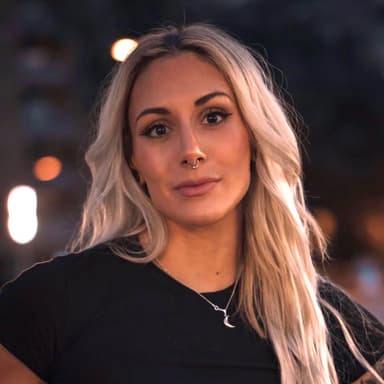
Dr Ana Rojo-Echeburúa