![[object Object]](/_next/image?url=https%3A%2F%2Fcdn.sanity.io%2Fimages%2Fei1axngy%2Fproduction%2Ff83f8e24d1fb84d1211763941d7874ed6b2a2da8-281x285.jpg&w=256&q=75)
by Prof Tim Dodwell
Updated 20 February 2023
Learning AI/ML to make a positive impact on sustainability: 11 sectors
![[object Object]](/_next/image?url=https%3A%2F%2Fcdn.sanity.io%2Fimages%2Fei1axngy%2Fproduction%2F3151b9a0f6458c94167e3f8b6fb84c801e272f2e-600x306.jpg&w=2048&q=75)
In the words of Billy Joel, "We didn't start the fire". The sustainability crisis has been brewing for decades. But now, advances in AI/ML mean we have the technology and tools to start putting the fire out - across multiple sectors.
In this article, we will look at 11 sectors where AI/ML can help to accelerate the sustainability mission. Global leaders like the UN have already established ambitious aims, such as the 17 Sustainable Development Goals (SDGs). Using data will enable us to understand the world's problems better and identify the solutions sooner. This is exactly what we do at digiLab: use AI/ML to tackle sustainability challenges. We’re helping to make nuclear fusion a reality by working with the UK Atomic Energy Authority, paving the way for clean energy. We’ve worked with water utility companies like South West Water, to reduce the chemical pollution in rivers. And we’re partnering with organisations like NATS to usher in the future of airspace management.
By acquiring a solid foundation in Artificial Intelligence and Machine Learning, you too could do more than just improve algorithms for social media feeds. You could make a difference in a variety of sectors, with job titles like Environmental AI Developer, Ethical Robotics Engineer, Emissions Trader, Decarbonisation Advisor, or Supply Chain Reporting Specialist.**
Which of the following sectors is the most interesting to you?
1. Traffic Management: reducing congestion and therefore overall fuel burn I always sit in traffic and wonder, "Why here? Why now?". It's not always a case of being unlucky, of being in the wrong place at the wrong time. Although some congestion is caused by unavoidable black swan events - e.g. a crash - lots of congestion is due to bad road design or traffic management. Check out this simulator to see how jams form in real-time by themselves.
AI/ML can help solve these systematic issues. It can monitor live data feeds from transport infrastructure, and then recommend system updates, such as lane control, alternative speed limits, traffic light co-ordination. It can also help urban planners design new networks or update existing ones.
2. Manufacturing: optimising processes to minimise energy consumption and waste Everyone loves win-wins. Reducing energy consumption and waste is a implementation of AI/ML which benefits an organisation's bottom line AND the planet's top line! In other words, avoidable natural resource depletion is also an avoidable cost.
3. Building Management: adjusting lighting and heating based on occupancy patterns ESG investing has really taken off in the last few years, and companies are feeling the heat (finally a good fire!). One easy way for them to improve their environmental credentials is by making their real estate footprint greener, and landlords are having to listeng. AI systems provide a highly scalable method for automating much of the day-to-day temperature and lighting control, by analysing data on e.g. occupancy levels. Because we all forget to turn the lights off...
4. Recycling Solutions: object detection to improve recycling separation Think putting things in the recycling bin means that they'll be recycled? Not so fast. Many waste disposal facilities still rely on individual workers to identify what's actually recyclable, so lots of items get missed or miscategorised. Humans aren't perfect... Nor are machines, but at least they don't get tired as quickly! Object detection, a form of ML, can massively improve the sorting hit rate.
5. Weather Prediction: enabling organisations to proactively respond to extreme events It's not just fires. It's also hurricanes and floods. These extreme events are increasing in frequency. We collect lots of weather data, but until it's a really obvious thing, humans aren't great at predictions. AI/ML can help. By anticipating extreme events sooner, we can put out more accurate warnings, place less stress on disaster relief teams, and ultimately save more lives.
But when the weather report says it will be sunny today - and you live in Britain - you can still expect "unexpected" showers in the afternoon.
6. Renewables Monitoring: improving uptime and efficiency for wind and solar farms Unsurprisingly, not many engineers WANT to live on off-shore wind farms. But things do break when no-one's looking. Machine Learning systems can begin to identify early signs of e.g. component stress, and therefore can alert repair teams if they need to head out to the farm and make pro-active repairs. This means better uptime, and a more sustainable energy mix.
7. Sustainable Agriculture: minimising the use of pesticides and fertilisers and improving the efficiency of irrigation systems We need to produce more food! We need to use less pesticide! Agriculture = puzzled.
Over the past decades, we've managed to massively increase agricultural productivity, but we've had to apply vast amounts of harmful chemicals. This isn't sustainable. AI/ML can help to drive productivity gains by harnessing the power of data alone.
8. Protecting Biodiversity: tracking flora and fauna to assist conservation efforts This is a particularly exciting sector for any budding Attenborough who also love data! This Guardian article outlines five fascinating ways that AI/ML is helping to save wildlife. Our favourite is protecting koalas, by using AI to analyse infrared drone footage and identify if koala communities are in danger.
9. Modelling Settlements: enabling more liveable, earth-friendly cities and infrastructure AI/ML is helping to take the guesswork out of urban planning. Firstly, we can unlock hidden insights in the data being generated from current settlements, to discover the root causes of urban pain points. Secondly, we can use digital twins to model changes to existing settelements or hypothesise the best way to build new settlements. Because as complex as Machine Learning is, it's not quite as much work as building a skyscraper. Usually.
10. Sustainable Supply Chains: identifying supply chain risks and bad actors Supply chains connect raw items with the things we use. They can involve multiple suppliers, multiple manufacturers, multiple distributors, multiple retailers. Organisations "downstream" are keen to avoid the reputational scandals of sourcing from bad actors "upstream". But they don't have an infinite number of people working in procurement and compliance. AI can reduce the workload by flagging potential risks for further inspection.
11. Optimising Logistics: reducing the time polluting vehicles are on the road, or in the air, or navigating the seas... AI/ML, meet the travelling salesman problem (and it's various extensions!). Reader, get ready for another win-win. If logistics operators are more efficient, then businesses spend less on fuel and other transport costs; consumers get products quicker; the planet doesn't suffer from as much pollution! So you can order your next grocery delivery to your home, guilt free.
Are you passionate about one of these sectors?
We’ve designed our first course, AI in the Wild: Foundations in Machine Learning, to enable beginners to land their first job in these sorts of sectors. We’d like more people to use AI/ML in their jobs – we’d LOVE more people to use AI/ML in tackling real-world problems!
AI/ML is proving itself to be more and more of an essential tool in tackling sustainability challenges, such as improving energy efficiency, enhancing waste management, promoting sustainable agriculture, protecting biodiversity, and reducing supply chain risks. Want to make a difference yourself? Get started with the digiLab Academy!
About digiLab
digiLab is an agile, deep tech company operating as a trusted partner to help industry leaders make actionable decisions through digital twin solutions. We invent first-of-a-kind, world-leading machine learning solutions which empower senior decision-makers to exploit the value locked in their data and models. The digiLab Academy is how we distil this knowledge into beginner-friendly courses.
Featured Posts
If you found this post helpful, you might enjoy some of these other news updates.
Python In Excel, What Impact Will It Have?
Exploring the likely uses and limitations of Python in Excel
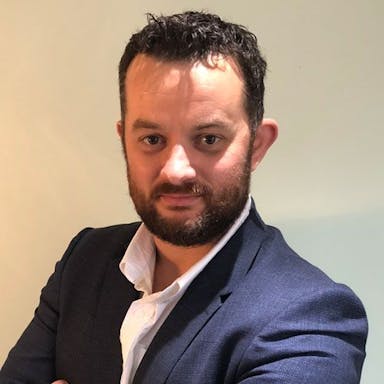
Richard Warburton
Large Scale Uncertainty Quantification
Large Scale Uncertainty Quantification: UM-Bridge makes it easy!
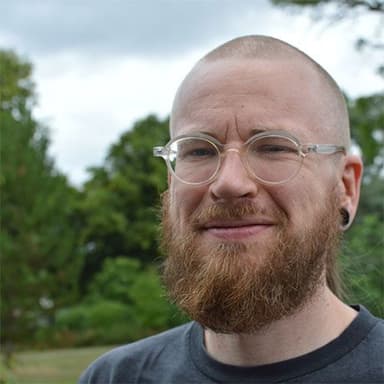
Dr Mikkel Lykkegaard
Expanding our AI Data Assistant to use Prompt Templates and Chains
Part 2 - Using prompt templates, chains and tools to supercharge our assistant's capabilities
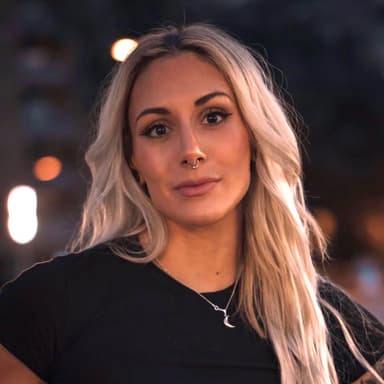
Dr Ana Rojo-Echeburúa