![[object Object]](/_next/image?url=https%3A%2F%2Fcdn.sanity.io%2Fimages%2Fei1axngy%2Fproduction%2Fe21406046c81774a7958931b9d4156cf07de0987-460x460.jpg&w=256&q=75)
by Dr Mikkel Lykkegaard
Updated 26 September 2023
senSiteUQ: A New Paradigm for Sewer Network Monitoring
![[object Object]](/_next/image?url=https%3A%2F%2Fcdn.sanity.io%2Fimages%2Fei1axngy%2Fproduction%2Fa144976881b2ed5ac3ab91dffa10ff0750fd1232-912x693.png&w=2048&q=75)
The water sector is currently installing sensors in sewer networks across the globe. In the UK alone, it is expected that water companies will install more than 300,000 sensors by 2030. This is partly driven by regulation, but is enabled by dramatic drop in the price of sensors that opens up for more efficient and controled operations of sewer systems. A monitored sewer system provides the means to improve many key objectives, including forecasting and preventing CSO overflows, identifying blockages, identifying the source of sewer contamination, predictive maintanance, etc. This transition of equipping the sewer system with sensors is an enormous and extremely costly undertaking.

Deciding how many sensors to purchase and where to put them is currently heuristically driven. The cost of sensors, physical constraints such as the ease of access and IOT connectivity, and expert opinion all factor in, but the heuristic approach does not allow for measurable detection targets and quantitative requirements. In contrast, senSiteUQ places sensors based on a rigorous, scientific approach that ensures that each sensor contributes the most possible information about the network.
Optimal Sensor Placement for Sewer Networks
Optimal experimental design or optimal sensor placement, are two perspectives of the same underlying problem. How can we reduce the number of experiments or sensors without sacrifing confidence in our results?
The research on the topic includes both mature methods such as "traditional" optimal design (see Goos & Jones, 2011) and sophisticated response-surface methods (see Garnett, 2023). While these methods are highly generalised and can be applied to virtually any problem, their relevance to practical engineering problems, such as the placement of sensors in a sewer network, is not obvious. Additonally, much of the literature on the topic is impenetrable to non-statisticians.

senSiteUQ offers an easy-to-use, cloud-based software application for the optimal placement of sensors in drainage networks. It integrates high-level information, including sewer network models (InfoWorks, MIKE+, SWMM), weather data, surface conditions and IOT connectivity. Sensor placement designs can be targeted to forecast CSO overflow, identify blockages, perform predictive maintencance, or a combination of all of the latter. It is being developed in close collaboration with industry partners to ensure alignment with industry needs.
The illustration below shows an example design for the benchmark sewer network Astlingen (see Schütze et al., 2018). Here, the design was chosen to monitor the hydraulic behaviour of central, critical nodes in the system which are expected to have a high impact on the performance of the sewer network.

Placing Sensors with Confidence
Monitoring a sewer system adequately is a complex and challenging task. Placing sensors is the first step towards a robust decision support system, but the sensor placement strategy can make or break this system. Sensor placement typically relies on a variety of data which, which is chosen and assessed by an expert. While this can certainly result in good placement strategies, the efficacy of a given strategy is neither quantifiable nor tracable.
senSiteUQ provides an alternative, rigorous approach to sensor placement in sewer networks, where the decisions that determine the placement strategy are entirely transparent and traceable. It is fully automatic and reduces the number of required sensors. This improves the current approach on multiple fronts: by reducing the cost of monitoring a sewer network, by simplifying the auditing of plans and proposals, and by reducing the workload for the sensor deployment team.
senSite Features
- Simulation-driven or simulation-free optimal sensor placement.
- Integration of geospatial data, such as IOT connectivity and surface conditions.
- Multi-objective optimisation for blockage detection, CSO overflow forecasting and contaminant source identification.
- Compatibility with industry standard modelling tools, such as InfoWorks, MIKE+, and SWMM.
- Simple and intutive interface, made for practitioners.
References
Goos, P., & Jones, B. (2011). Optimal design of experiments: A case study approach. Wiley.
Garnett, R. (2023). Bayesian optimization. Cambridge University Press.
Schütze, M., Lange, M., Pabst, M., & Haas, U. (2018). Astlingen – a benchmark for real time control (RTC). Water Science and Technology, 2017(2), 552–560.
Featured Posts
If you found this post helpful, you might enjoy some of these other news updates.
Python In Excel, What Impact Will It Have?
Exploring the likely uses and limitations of Python in Excel
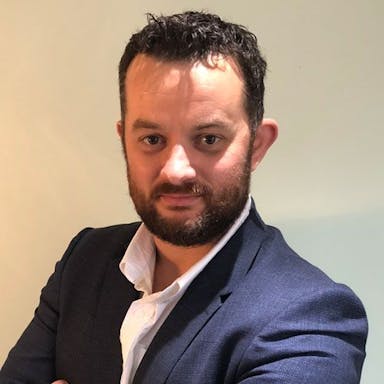
Richard Warburton
Large Scale Uncertainty Quantification
Large Scale Uncertainty Quantification: UM-Bridge makes it easy!
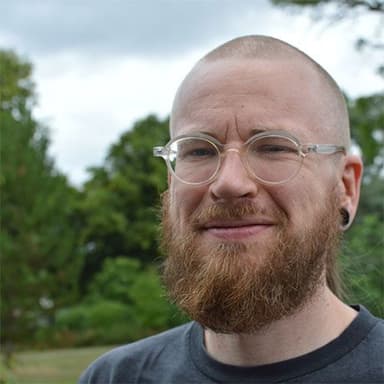
Dr Mikkel Lykkegaard
Expanding our AI Data Assistant to use Prompt Templates and Chains
Part 2 - Using prompt templates, chains and tools to supercharge our assistant's capabilities
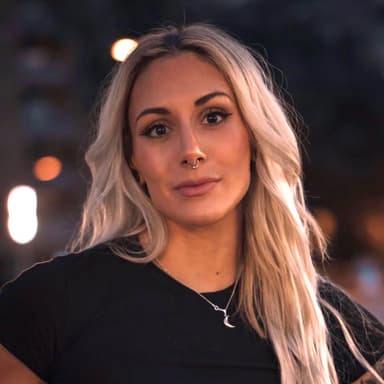
Dr Ana Rojo-Echeburúa