![[object Object]](/_next/image?url=https%3A%2F%2Fcdn.sanity.io%2Fimages%2Fei1axngy%2Fproduction%2Fcc5ab2d63daeea476490218d4e41b58074e8d535-2147x2147.jpg&w=256&q=75)
by Dr Alexander Mead
Updated 26 September 2023
Inverse Problems in Science and Engineering
![[object Object]](/_next/image?url=https%3A%2F%2Fcdn.sanity.io%2Fimages%2Fei1axngy%2Fproduction%2F2515ad820c684a5bb0bd2a371bc7b3314e622c60-1616x752.png&w=2048&q=75)
Professionals and researchers in the fields of science and engineering often encounter challenges that require the use of unconventional problem-solving methods. One such framework that has proven increasingly useful is the use of "inverse methods" to solve "inverse problems". Although inverse problems are studied and solved by statisticians and computer scientists, inverse problemscare ubiquitous and appear in all scientific and engineering fields. This article aims to demystify the essence of inverse problems and underline their ubiquity to real-world applications.
Understanding the Concept of an Inverse Problem
"Inverse" problems can be understood as questions where the outcome is known, but the conditions that led to it are not. "Forward" problems, in contrast, are where initial conditions are known, and the objective is to predict the outcome.
Forward problems generally occur in the direction of the flow of time, making them amenable to physics-based simulation; finite element or hydrodynamic simulations can model everything from stresses on aircraft wings to galaxy formation. The inverse problem usually involves some inference about what must have happened in the past, which is usually either difficult or impossible to simulate directly. In addition, more than one set of parameters may have led to very similar or identical observations, and we want to be able to quantify the uncertainty over the parameters that may have led to the outcome we observed.
Examples
The concept of an inverse problem transcends traditional disciplinary boundaries:
Engineering: System Identification and Control
In control systems engineering, the behaviour of a system often needs to be understood based on output data. For example, in an aircraft control system, engineers might have data on the response of a plane to various control inputs. The inverse problem is to estimate the aerodynamic characteristics and system parameters that resulted in those responses, which can be crucial for improvements in design and safety.
Healthcare: Medical Imaging
Medical imaging technologies like Magnetic Resonance Imaging (MRI) and Computed Tomography (CT) scans are archetypical examples. In these techniques, the instrument collects data, such as radiation absorption or magnetic field variations, and an algorithm solves an inverse problem to reconstruct a detailed internal image of the body. Accurate solutions to these inverse problems are crucial for diagnosis and treatment planning.
Earth Sciences: Seismology
In seismology, scientists often deal with inverse problems when interpreting data from seismic surveys. When an earthquake generates seismic waves that travel through the Earth sensors pick up these waves at various locations. The inverse problem is to use this wave data to map out the geological structures inside the Earth — a critical ingredient for understanding natural disasters, resource exploration, and environmental studies.
How inverse methods are used at digiLab
In engineering applications, it is common to attempt to invert models using direct numerical techniques such as root finding via Newton's method. Essentially values of the parameters corresponding to the observation are guessed, and then this guess is sequentially improved on until some level of accuracy is achieved. This method can work, but convergence might be slow and difficult with noisy data. Furthermore, any uncertainty about the parameter values that might have led to the observation is not captured.
Using machine learning to create a surrogate model can reduce the time and expense of solving inverse problems and in some cases, it can make solving an impossible problem, possible. At digiLab, we have a unique approach to solving inverse problems. We have improved on known algorithms such as Markov Chain Monte Carlo (MCMC) to quantify uncertainty and solve the problem faster.
Case study: Tritium desorption in fusion-reactor walls
An example of an inverse problem that digiLab has worked on involves the desorption (the physical processes by which a previously absorbed substance is released from a surface) of tritium nuclei from the walls of a fusion reactor. The forward model (which we want to invert) is a simulation of the desorption process given different assumptions about reactor-wall material properties. The simulation calculates a temperature-dependent desorption rate. The inverse problem to be solved is: "Given some (noisy) measurements of the desorption rate, what can we learn about the properties of the reactor wall?"

Fig. 1
In Fig. 1 we see the results of a solution to an inverse problem. The (blue) crosses denote measurements of the desorption rate across a range of temperatures. These are the observed data. We would like to know the range of reactor-wall properties that could have led to these observations. Each curve shows a temperature-dependent desorption rate for a specific reactor-wall model, and these are coloured by how probable each model is. Solving the inverse problem not only tells us the most-likely reactor wall that led to our observations (the darked curve), but also the distribution of possible reactor walls that may have led to the observations.
Conclusion
It is worth emphasising that inverse problems may have multiple solutions, especially when dealing with real-world, noisy data. While the complexities of solving these problems can be daunting, the advancements that digiLab has made in techniques such as Markov Chain Monte Carlo (MCMC) algorithms are making it increasingly manageable to derive robust solutions with reliably quantified uncertainty.
The notion of an inverse problem offers a profound yet pragmatic framework for addressing a multitude of challenges in science and engineering. While the mathematics behind solving these problems can become intricate, a foundational understanding can significantly enrich problem-solving capabilities. Whether it is diagnosing medical conditions, exploring subterranean geological formations, or optimizing complex engineering systems, a knowledge of inverse problems and how to solve them is an invaluable tool in modern scientific inquiry.
Interested in solving an inverse problem with twinLab?
Featured Posts
If you found this post helpful, you might enjoy some of these other news updates.
Python In Excel, What Impact Will It Have?
Exploring the likely uses and limitations of Python in Excel
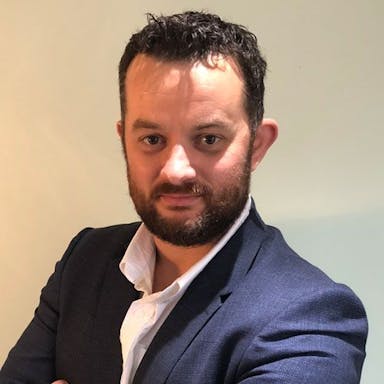
Richard Warburton
Large Scale Uncertainty Quantification
Large Scale Uncertainty Quantification: UM-Bridge makes it easy!
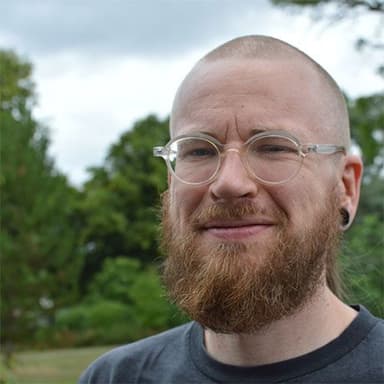
Dr Mikkel Lykkegaard
Expanding our AI Data Assistant to use Prompt Templates and Chains
Part 2 - Using prompt templates, chains and tools to supercharge our assistant's capabilities
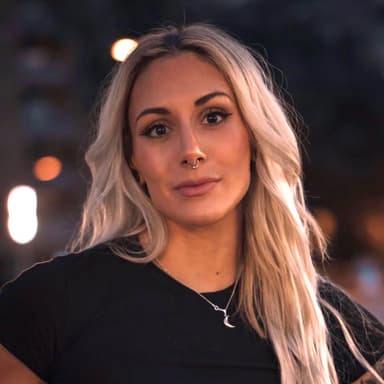
Dr Ana Rojo-Echeburúa